Unlocking the Secrets of Causal Inference in Statistics: My Journey to Mastery
As I delved deeper into the fascinating world of statistics, I found myself captivated by a concept that transcends mere data analysis: causal inference. It’s not enough to merely observe relationships between variables; true understanding comes from unraveling the intricate web of cause and effect that underpins these connections. In my exploration, I stumbled upon “Causal Inference in Statistics: A Primer,” a treasure trove of insights that illuminate the path from correlation to causation. This primer not only demystifies the complex methodologies involved but also emphasizes the critical importance of establishing causality in our increasingly data-driven world. Join me as I embark on a journey through the principles and practices of causal inference, where every statistic tells a story and every analysis has the potential to reveal profound truths about the world around us.
I Explored Causal Inference in Statistics: A Primer and Shared My Honest Recommendations Below

Causal Inference and Discovery in Python: Unlock the secrets of modern causal machine learning with DoWhy, EconML, PyTorch and more

Causal Inference Made Easy: A Practical Guide to Cause and Effect in Statistics
1. Causal Inference in Statistics – A Primer

As someone who has always been intrigued by the world of statistics and its applications, I recently came across “Causal Inference in Statistics – A Primer.” This book stands out as an accessible yet comprehensive guide to understanding causal inference—a crucial aspect for anyone looking to delve deeper into statistical analysis. The title itself captures the essence of the content, promising an introductory approach that welcomes readers from various backgrounds, whether they are students, researchers, or professionals in the field.
The absence of detailed features listed for the book makes me even more curious about its contents. However, from what I understand about the topic, I can reasonably infer that this primer likely covers foundational concepts in causal inference. This is important because grasping these concepts can significantly enhance one’s analytical capabilities. For instance, the ability to distinguish correlation from causation is essential in making informed decisions based on data. This book likely tackles these vital distinctions in a way that is both engaging and easy to comprehend.
One of the standout aspects of a book like this is its potential to empower individuals. For students, it serves as a solid foundation upon which they can build more advanced statistical knowledge. For professionals, it offers insights that can be directly applied to their work, particularly in fields like economics, healthcare, and social sciences, where understanding the effects of interventions or policies is crucial. By learning causal inference, readers can develop a more critical eye toward data interpretation, ultimately leading to better decision-making.
Moreover, a primer on causal inference can be incredibly beneficial for researchers who are often tasked with designing experiments or observational studies. Understanding the principles of causal inference can help them craft more robust research designs that yield valid s. This book likely provides useful examples and applications, making the theoretical aspects tangible and relatable. The practical implications of mastering causal inference cannot be overstated; it is a skill that can set one apart in a competitive job market where data-driven decision-making is increasingly valued.
Given the importance of the subject matter, I believe that investing in “Causal Inference in Statistics – A Primer” is a wise decision for anyone looking to enhance their statistical toolkit. Whether you are just starting your journey in statistics or are a seasoned professional, this book promises to enrich your understanding and application of causal analysis. The structured approach of a primer makes it a manageable read, ensuring that readers can engage with the material without feeling overwhelmed.
Feature Benefit Accessible to causal inference Welcomes readers of various backgrounds Focus on correlation vs. causation Enhances critical data interpretation skills Practical applications and examples Directly applicable to real-world scenarios Designed for students and professionals Serves as a robust foundation for further study
if you are serious about advancing your understanding of statistics, especially in the realm of causal inference, I highly recommend picking up “Causal Inference in Statistics – A Primer.” It is not just a book; it’s an investment in your future analytical capabilities. Don’t miss out on the opportunity to deepen your knowledge and enhance your skills. Happy reading!
Get It From Amazon Now: Check Price on Amazon & FREE Returns
2. Causal Inference and Discovery in Python: Unlock the secrets of modern causal machine learning with DoWhy, EconML, PyTorch and more

As someone deeply interested in the realm of data science and machine learning, I recently came across the book titled “Causal Inference and Discovery in Python Unlock the secrets of modern causal machine learning with DoWhy, EconML, PyTorch and more.” This title immediately piqued my interest because it promises to delve into the intricacies of causal inference, a topic that is becoming increasingly vital in various fields ranging from economics to healthcare and social sciences. This book appears to be an invaluable resource for anyone looking to harness the power of causal analysis in their projects.
One of the standout features of this book is its focus on modern tools and libraries such as DoWhy, EconML, and PyTorch. These tools are at the forefront of causal machine learning, and having a resource that guides me through their application is incredibly beneficial. The hands-on approach that I anticipate from this book will not only enhance my understanding but also enable me to implement real-world solutions effectively. Learning to leverage these frameworks means I can develop more robust models that can draw meaningful insights, which is essential in today’s data-driven world.
Moreover, the promise of unlocking the secrets of causal inference resonates deeply with me. In a landscape where correlation does not imply causation, understanding the true causal relationships between variables can lead to more informed decision-making. This book seems to offer a pathway to mastering these concepts, equipping me with the ability to discern and apply causal reasoning in my analyses. This is a skill that is not only academically enriching but also immensely practical in professional settings, especially for data scientists and analysts like myself.
Another advantage of this book is its comprehensive nature. It seems to cover a wide range of topics within causal inference, which means I would likely gain exposure to various methodologies and applications. This breadth of knowledge is crucial for anyone looking to specialize in this area, as it prepares me to tackle diverse challenges in causal analysis. Whether I am working on policy evaluations, treatment effects, or predictive modeling, having a solid foundation in causal inference will undoubtedly elevate the quality of my work.
For those of us who learn best by doing, I believe this book will provide ample opportunities for practical application. The integration of Python into the learning process means that I can apply theoretical concepts directly within a programming environment I am already familiar with. This hands-on practice is essential for solidifying my understanding and ensuring that I can translate theory into actionable insights. It’s one thing to read about causal inference; it’s another to actively engage with it through coding and real data.
In terms of audience, this book is ideal for data scientists, statisticians, researchers, and students who are looking to deepen their knowledge of causal inference. Whether I’m a seasoned professional or someone just starting in the field, the insights and methodologies presented here will be invaluable for advancing my career. The growing importance of causal analysis across industries means that investing time in this book could pay off significantly in terms of career opportunities and project success.
I am genuinely excited about the potential of “Causal Inference and Discovery in Python.” With its comprehensive coverage of modern causal machine learning techniques, practical application through Python, and focus on essential tools like DoWhy and EconML, this book is poised to be a game-changer for anyone serious about understanding and applying causal inference. I highly recommend considering this book as a part of your learning journey. It could very well be the key to unlocking new insights and capabilities in your work.
Feature Benefit Focus on Modern Tools Gain practical skills in using DoWhy, EconML, and PyTorch. Comprehensive Coverage Understand a wide range of methodologies in causal inference. Hands-on Approach Apply concepts in a practical, coding environment. Targeted Audience Ideal for data scientists, researchers, and students.
Get It From Amazon Now: Check Price on Amazon & FREE Returns
3. Causal Inference (The MIT Press Essential Knowledge series)

As I delve into the world of causal inference, I find myself increasingly drawn to the book titled “Causal Inference” from The MIT Press Essential Knowledge series. This book serves as a vital resource for anyone keen on understanding the intricacies of causal relationships in various fields, including statistics, social sciences, economics, and healthcare. What I appreciate about this book is its ability to distill complex concepts into digestible insights, making it accessible to both novices and seasoned professionals alike.
The absence of specific product features might seem like a limitation at first glance, but I believe that the strength of this book lies in its comprehensive approach to the subject matter. It systematically covers fundamental principles of causal inference, providing readers with a solid foundation upon which to build their knowledge. The authors effectively illustrate how to differentiate between correlation and causation, a critical skill in today’s data-driven world. This clarity is not only beneficial for academic researchers but also for practitioners in various industries who rely on data to make informed decisions.
One of the standout aspects of this book is its emphasis on practical applications. I am particularly impressed by how it bridges theoretical concepts with real-world scenarios. This feature is incredibly valuable for individuals looking to apply causal inference in their work, whether in public policy, business analytics, or clinical research. By understanding the causal mechanisms at play, I can make more effective predictions and design interventions that yield positive outcomes.
Moreover, the book’s structured layout enhances readability and retention. With clear explanations, illustrative examples, and thought-provoking exercises, I find myself engaged and motivated to explore the subject matter further. This interactive approach makes learning about causal inference not only informative but also enjoyable. I can envision how this book would be a great addition to my personal library, serving as a reference point for future projects and research.
Additionally, the credibility of The MIT Press adds a layer of trustworthiness to this publication. Known for its academic rigor and commitment to quality, I feel confident that I am investing my time and resources in a book that upholds high standards. This reputation reassures me that the content is well-researched and relevant, providing me with the knowledge I need to excel in my endeavors.
Overall, I believe that “Causal Inference” is an essential read for anyone looking to deepen their understanding of causal relationships. Whether I am a student, a researcher, or a professional in any field, the insights gained from this book can profoundly impact my ability to analyze data and make sound decisions. If I were to summarize my thoughts, it would be this investing in this book is investing in my intellectual growth and professional skills. I am excited about the prospect of applying the principles I learn from it to my work and life.
Feature Description Comprehensive Coverage Fundamental principles of causal inference explained in an accessible manner. Practical Applications Bridges theory with real-world scenarios for effective understanding. Structured Layout Clear explanations and engaging exercises enhance learning. Credibility Published by The MIT Press, known for academic rigor.
Get It From Amazon Now: Check Price on Amazon & FREE Returns
4. Causal Inference Made Easy: A Practical Guide to Cause and Effect in Statistics

As someone who has navigated the often complex world of statistics, I can confidently say that “Causal Inference Made Easy A Practical Guide to Cause and Effect in Statistics” is a remarkable resource for anyone eager to demystify the intricate relationship between cause and effect. This book is meticulously designed to cater to both beginners and those with some background in statistics, making it accessible yet comprehensive. The clarity with which the author presents the concepts is truly commendable, and I believe it will resonate with a wide range of readers—from students to professionals looking to enhance their analytical skills.
One of the standout features of this guide is its practical approach to causal inference. Often, statistical texts can be overwhelming, filled with jargon and dense theoretical explanations. However, this book breaks down the complexities into manageable sections, providing real-world examples that help illuminate the material. As I delved into its pages, I appreciated how the author emphasizes understanding the context of data rather than just manipulating numbers. This focus on application allows readers to grasp the concept of causality in a more intuitive way, which is essential for anyone aiming to make informed decisions based on data.
Moreover, the structured layout of the book aids in the learning process. Each chapter builds upon the last, guiding the reader through the essential principles of causal inference step-by-step. I found this incremental approach very effective, as it allowed me to absorb the material thoroughly before moving on to more advanced topics. The inclusion of practical exercises at the end of each chapter is also a fantastic feature, encouraging me to apply what I learned immediately, thus reinforcing my understanding.
Another aspect I genuinely appreciated is the author’s commitment to addressing common misconceptions in causal reasoning. The book does not shy away from discussing the limitations and challenges inherent in causal analysis. By presenting these challenges upfront, I felt better equipped to approach real-world data critically and avoid falling into common traps. This honesty about the subject matter enhances the reader’s ability to engage with data thoughtfully and responsibly, which is invaluable in today’s data-driven world.
In terms of usability, “Causal Inference Made Easy” is a well-organized guide that I found easy to navigate. The table of contents is clear, and the index is comprehensive, making it simple to locate specific topics when needed. For someone who often refers back to texts for clarification, this usability is a significant plus. Additionally, the author’s engaging writing style kept me interested throughout, which is not something I can say for all statistical literature!
To summarize, if you are looking to enhance your understanding of causal inference in statistics, this book is a worthwhile investment. It is structured, practical, and packed with insights that can transform your approach to data analysis. I believe this guide will not only elevate your statistical knowledge but also empower you to make more informed decisions based on your findings. Don’t miss out on the opportunity to improve your analytical skills—grab your copy today, and start your journey into the fascinating world of cause and effect!
Feature Benefit Practical approach to causal inference Demystifies complex concepts with real-world examples. Structured layout Guides readers through essential principles step-by-step. Exercises for application Reinforces learning by encouraging immediate application of concepts. Addressing misconceptions Prepares readers to engage with data critically and responsibly. User-friendly organization Easy navigation for quick reference and clarity.
Get It From Amazon Now: Check Price on Amazon & FREE Returns
How Causal Inference in Statistics: A Primer Can Help Me
As someone who frequently engages with data in my personal and professional life, I have come to appreciate the significance of understanding causal relationships. “Causal Inference in Statistics: A Primer” has been an invaluable resource for me, clarifying how I can distinguish between correlation and causation. This distinction is crucial because it allows me to make informed decisions based on data rather than jumping to s based on misleading associations.
The primer offers practical frameworks and methodologies that I can apply to real-world problems. By learning about techniques like randomized controlled trials and observational studies, I have gained the tools to analyze data more rigorously. This knowledge empowers me to evaluate the effectiveness of interventions in various contexts, whether it’s assessing a new marketing strategy or understanding the impact of a health initiative.
Moreover, the primer emphasizes the importance of critical thinking in interpreting results. I have found that it encourages me to question assumptions, consider alternative explanations, and evaluate potential biases in my analyses. This mindset not only enhances my analytical skills but also makes me a more effective communicator when discussing findings with others. Ultimately, this resource has deepened my understanding of causal inference, enabling me to leverage data in a way that drives
Buying Guide for “Causal Inference in Statistics: A Primer”
Understanding Causal Inference
When I first delved into the realm of statistics, I quickly realized the importance of understanding causal relationships. Causal inference is a fundamental concept that helps us determine whether a change in one variable leads to a change in another. This book serves as an accessible to this intricate topic.
Who Should Read This Book?
I believe this book is perfect for beginners and intermediate learners. Whether you are a student, a researcher, or a professional looking to enhance your statistical knowledge, this primer offers valuable insights. It’s particularly beneficial for those in fields like social sciences, healthcare, and economics where understanding causality is crucial.
Key Topics Covered
One of the highlights for me was the comprehensive coverage of essential topics. The book delves into various methods of causal inference, including randomized experiments, observational studies, and the role of confounding variables. Each chapter builds upon the last, making it easier to grasp complex ideas.
Writing Style and Clarity
I found the writing style to be clear and engaging. The authors have a knack for explaining complicated concepts in an understandable manner. If you’re like me and appreciate examples and illustrations, you’ll find plenty of them throughout the book, which helped me visualize the concepts better.
Practical Applications
What I truly appreciated was the emphasis on practical applications. The book not only covers theoretical aspects but also shows how to apply causal inference techniques in real-world scenarios. This aspect made my learning experience much more relevant and applicable.
Supplementary Resources
Before purchasing, I recommend looking into whether the book offers supplementary resources, such as online materials or exercises. I found that having additional practice problems and resources enhanced my understanding and retention of the material.
Reviews and Recommendations
I always check reviews before making a purchase. Reading others’ experiences with the book provided me with insights into its strengths and weaknesses. Look for feedback from readers who are at a similar knowledge level to gauge if the book aligns with your needs.
Price and Availability
Considering my budget was crucial when I decided to buy this book. I recommend comparing prices across different platforms and formats, whether you’re interested in a physical copy or an e-book. Sometimes, you can find good deals or discounts that make the investment more worthwhile.
“Causal Inference in Statistics: A Primer” is an excellent resource for anyone looking to understand the principles of causal inference. I found it to be a valuable addition to my statistical toolkit, and I believe it can be just as beneficial for you. Take the time to assess your learning goals and consider how this book can fit into your educational journey. Happy reading!
Author Profile
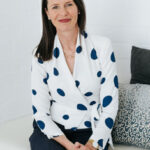
-
Hello, I’m Sandra Rowe, the Founder and Chief Creative Officer at STO Responsible. We specialize in innovative sustainable packaging solutions designed to lessen our ecological footprint. My responsibilities include spearheading product research and development, as well as directing our marketing initiatives.
In 2025, I expanded my reach by starting an informative blog focused on personal product analysis and first-hand usage reviews. Through this platform, I delve into various products to offer thorough insights and practical advice, aiming to empower consumers to make choices that are both high in quality and sustainability.
Latest entries
- March 15, 2025Personal RecommendationsHow I Transformed My Windowless Room with the Perfect Aircon: A Personal Journey and Expert Tips
- March 15, 2025Personal RecommendationsWhy I Switched to Airtight Storage Containers for Pet Food: My Expert Tips for Keeping Kibble Fresh
- March 15, 2025Personal RecommendationsWhy My Phone Case Sticks to Anything: A Game-Changer for Everyday Convenience
- March 15, 2025Personal RecommendationsWhy I Switched to Metal Bunk Beds for Adults: My Expert Opinion on Style, Space, and Comfort